Imagine robots that can navigate complex environments, grasp objects with precision, and perform delicate tasks – all without ever needing to be physically programmed. This is the ambitious goal of Nvidia’s generative physical AI, a revolutionary approach to robotics training that leverages the power of simulation.
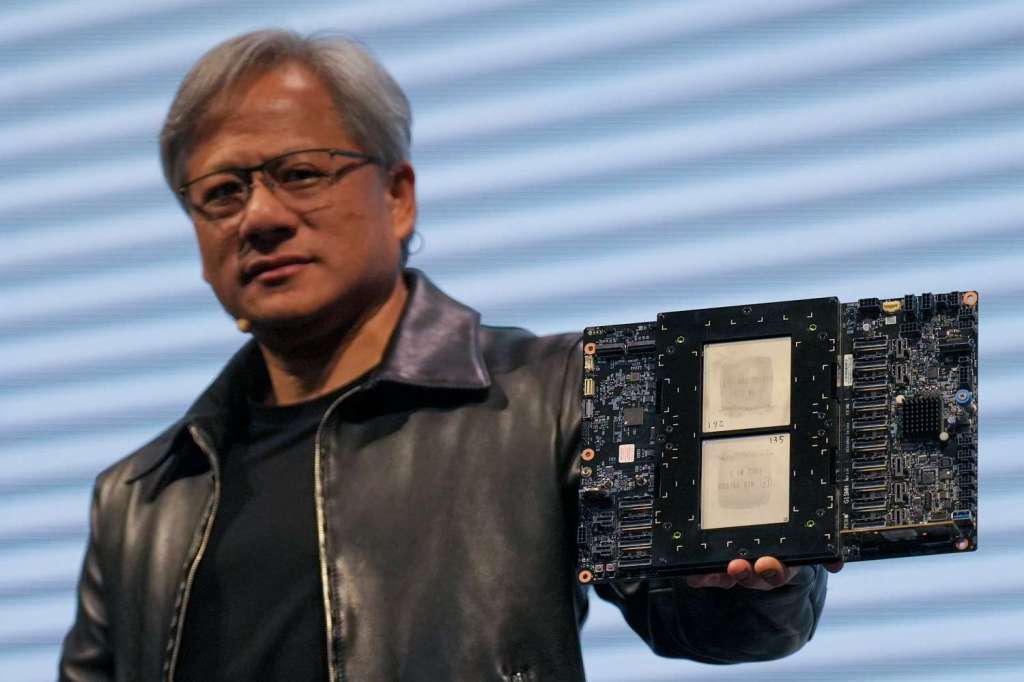
What is Generative Physical AI?
At its core, generative physical AI utilizes Nvidia’s Omniverse platform, a real-time 3D simulation environment. Within Omniverse, robots can be trained in meticulously crafted virtual worlds that mimic real-world scenarios. Here’s the technical magic behind it:
Physically Accurate Simulations: Omniverse employs physically based rendering and simulation techniques. This means that virtual objects in the simulation behave just as they would in the real world, taking into account factors like gravity, friction, and material properties. Imagine a virtual robot arm attempting to pick up a cup – the simulation will account for the cup’s weight, the slipperiness of its surface, and the robot arm’s movements to determine if the grasp is successful.
Generative AI for Learning: This is where it gets interesting. Nvidia incorporates generative AI techniques into the training process. Essentially, the AI can generate a vast number of realistic scenarios and challenges for the robots to navigate within the simulation. This allows the robots to learn through trial and error, constantly refining their movements and decision-making abilities in a safe and controlled virtual environment.
Benefits of Generative Physical AI:
Reduced Training Time and Costs: Traditionally, training robots involved extensive real-world testing, which can be time-consuming and expensive. Generative physical AI allows robots to learn a vast amount within the simulation, significantly reducing the need for physical training.
Improved Safety: Testing robots in the real world can be risky, both for the robot and its surroundings. Simulations eliminate this risk, allowing robots to experiment and learn from their mistakes without any physical consequences.
Enhanced Versatility: Generative AI can create an infinite variety of training scenarios within the simulation. This allows robots to be trained for a wider range of tasks and environments, making them more adaptable and versatile.
The Nvidia Stack
To power generative physical AI, Nvidia offers a comprehensive hardware and software stack:
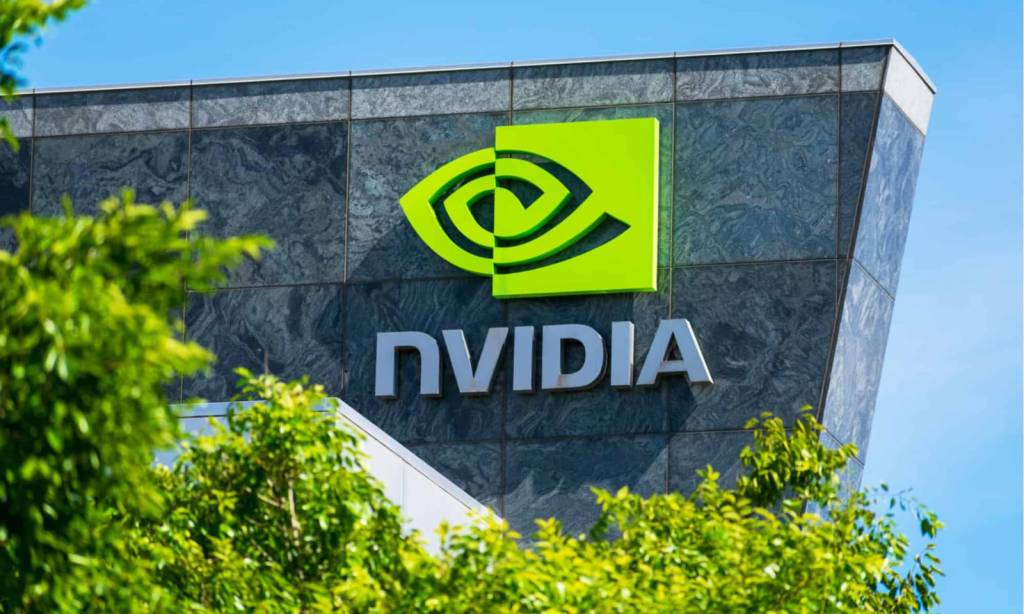
Nvidia AI Supercomputers: Training the complex AI models used in generative physical AI requires immense computational power. Nvidia’s AI supercomputers provide the necessary horsepower to handle these demanding tasks.
Jetson Orin and Thor: Once trained, the AI models need to be deployed on the robots themselves. Nvidia’s Jetson embedded systems, like the Orin and upcoming Thor, are specifically designed for running AI models on edge devices like robots.
Isaac Robotics Platform: This development platform provides all the tools and libraries needed to build and train robots using generative physical AI. It includes tools for simulating robots, creating realistic environments, and integrating AI models with robot hardware.
The Future of Robotics:
Nvidia’s generative physical AI represents a significant leap forward in robotics training. With the ability to learn and adapt in realistic simulations, robots are poised to become more capable, versatile, and efficient. This technology has the potential to revolutionize various industries, from manufacturing and logistics to healthcare and construction. As Nvidia continues to refine its generative physical AI technology, the future of robotics looks brighter than ever.
Leave a comment